P10-01
Decision-making model to enhance subjective well-being through individualized lifestyle modifications based on counterfactual explanation
Yunosuke MATSUDA *1, Satoshi WATANABE1, Yoji OKUGAWA1, Nobuyuki NAKANISHI1, Keishi MATSUMOTO1, Shinya HAYASAKA2
1BATHCLIN Corporation
2Faculty of Human Life Sciences, Tokyo City University
( * E-mail: matsuda_yuunosuke@bathclin.co.jp )
Subjective well-being (SWB), an individual's judgment about their overall well-being, is of broad interest, spanning both popular writing and scientific work. Some studies have reported that SWB is a multifaceted concept influenced by various factors, including economic stability, health, and personal growth. Numerous research reports have documented methods to enhance SWB, such as improving sleep quality. However, it is important to note that these methods are not universally applicable to all individuals. Therefore, we examined the feasibility of supporting individualized lifestyle modifications that increase SWB utilizing counterfactual (CF) explanation. CF explanation, a type of explainable machine learning technique, describes the changes needed in a sample to flip the outcome of the prediction. We developed a decision-making model based on 2022 survey data on lifestyle and quality of life, including an 11-point scale question on SWB. This decision-making model includes the SWB prediction model and employs CF explanation.
Using SHapley Additive exPlanations (SHAP) values for feature selection, we identified seven attributes (e.g., childhood memories of bathing, marital status, and household income) and seven lifestyle features (e.g., frequency of bathtub bathing, sleep duration, and the difference between bath time on weekdays and weekends) as effective predictors. These 14 features were used to train random forest regression for SWB prediction. Leave-one-out cross-validation was used to evaluate the model performance and generate CF explanations for each hold-out sample.
SHAP dependence plots calculated from the model tended to be consistent with previous research (e.g., predicted SWB peaked at approximately 7 hours of sleep duration and gradually increased with frequency of bathtub bathing from 0 to 4 per week). We obtained 859 CF explanations and found that frequency of bathtub bathing was included mainly. Focusing on CF explanations in the low frequency of bathtub bathing group, it was found that there was a difference depending on the sleep duration of instances. It was observed that some CF explanations recommended increasing not the frequency of bathtub bathing but the sleep duration for short sleepers (≤6 hours), even under low frequency of bathtub bathing conditions. Moreover, most CF explanations recommended healthy lifestyle modifications for short or long sleepers (≥8 hours) and instances of low frequency of bathtub bathing. Thus, this model allowed for both individualized support and previous standardized support.
In this study, CF explanations generated by our model were diverse and highly valid. However, the trained data include concerns over the impact on SWB by potentially unobserved variables and the lack of certainty in the direction of causality. Clarifying causal relationships or limiting the output of the model to effects observed in intervention studies would make the model more valuable and predictive.
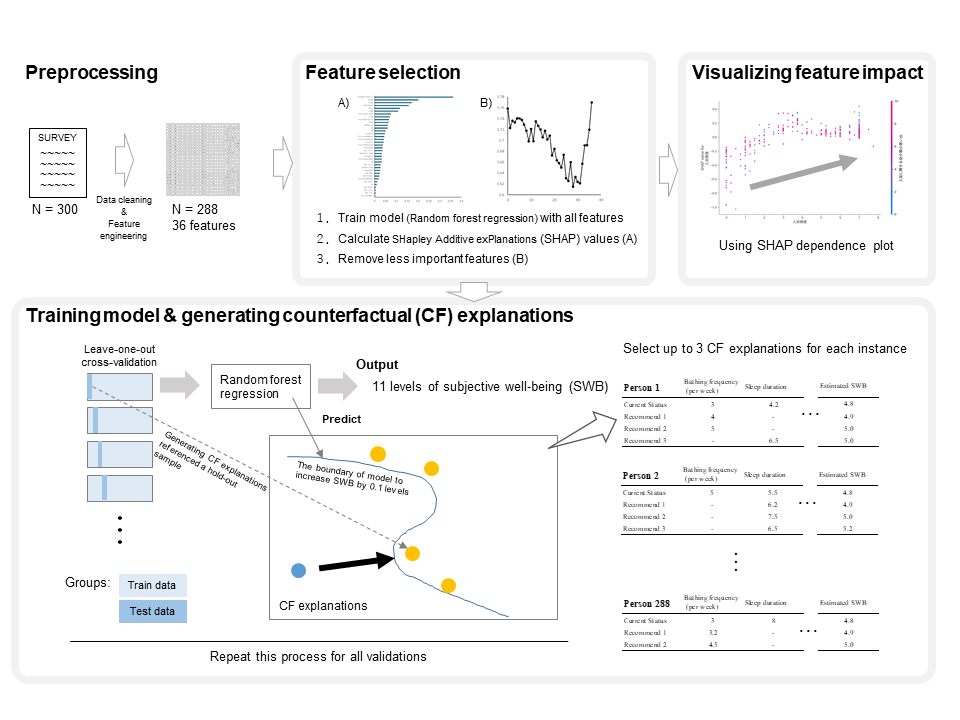