P07-21
Reaction-conditioned variational autoencoder model for catalyst generation and catalytic performance prediction
Apakorn KENGKANNA, Masahito OHUE
School of Computing, Institute of Science Tokyo
( * E-mail: kengkanna@li.c.titech.ac.jp )
Catalysts are materials that accelerate the rate of a chemical reaction without being permanently consumed. The structures of catalyst, particularly metal-ligand complexes, crucially determine the catalytic activity of chemical reactions. Designing effective catalysts is the key process for optimizing catalytic reactions. Recent approaches, including generative models, have been proposed to design new catalysts. However, these methods are developed for a specific reaction class without considering reaction components and conditions, and rely on predefined fragment categories, limiting novel discoveries outside the existing chemical space. Here, we present a reaction-conditioned generative model based on a variational autoencoder for generating catalysts and ligands and predicting their catalytic performance. This model is pre-trained on a large available reaction database, allowing for broader knowledge acquisition and fine-tuning for downstream reaction classes. The model shows comparable performance in catalytic activity prediction tasks, as well as demonstrates a promising way to generate possible catalysts and ligands for given reaction conditions. This work will help facilitate the optimization of ligands for catalysis and further enhance catalyst design and discovery processes in both the chemical and pharmaceutical industries.
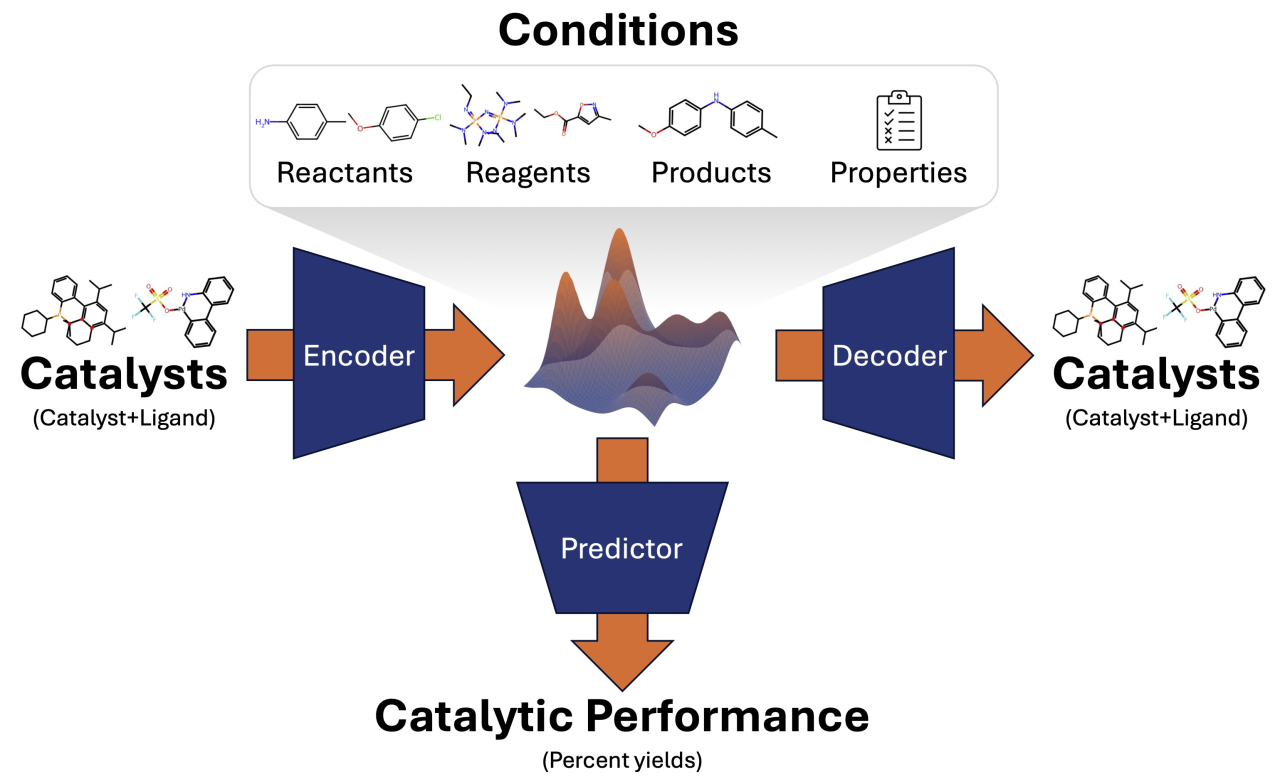