P03-29
A framework for enhanced de novo protein design using deep learning and bayesian optimization
Shuto HAYASHI *1, Jun KOSEKI2, Teppei SHIMAMURA1, 3
1Institute of Science Tokyo
2Cellular and Molecular Biotechnology Research Institute, National Institute of Advanced Industrial Science and Technology
3Nagoya University Graduate School of Medicine
( * E-mail: s-haya.csb@tmd.ac.jp )
The field of de novo protein design has experienced significant progress in recent years, particularly with the advent of deep learning techniques. These innovations have enhanced our ability to create custom-designed proteins for diverse applications, ranging from therapeutics to novel materials. However, despite these notable improvements, the functionality of computationally designed proteins often remains inferior to their naturally occurring counterparts or those developed through conventional expert-driven methodologies.
To address this problem, we introduce a design-build-test-learn (DBTL) cycle framework tailored for the development of proteins with enhanced functionality. The framework consists of two main phases: an initial pre-DBTL phase and an iterative DBTL cycle phase. In the pre-DBTL phase, we employ a combination of two deep learning-based protein design methods, specifically RFdiffusion and ProteinMPNN, to generate a diverse pool of potential functional proteins. To further enrich the diversity of the pool, we implement a combinatorial assembly strategy, which allows for the exploration of a broader sequence space.
Following the initial phase, highly functional proteins in the candidate pool are identified through the DBTL cycles, which comprise three key components: in silico evaluation, deep learning-based prediction, and multi-objective Bayesian optimization. During the in silico evaluation, we utilize MD simulations and MM/GBSA to assess protein characteristics, including binding free energies and structural stabilities. The results of the evaluation are then used to train an ensemble of neural networks. This ensemble model can be used not only to predict protein functionalities, but also to infer the uncertainty of the prediction, directly from amino acid sequences. Using the trained ensemble model as a surrogate model, we implement a multi-objective Bayesian optimization algorithm to propose promising protein candidates for subsequent rounds of evaluation.
To validate our framework, we applied it to the design of inhibitors targeting BCAT1, a key enzyme implicated in cancer cell metabolism. Our results demonstrate the efficacy of the pre-DBTL phase in generating proteins capable of inhibiting cancer cell proliferation. Furthermore, through the iterative DBTL cycle, we were able to identify proteins with substantially enhanced inhibitory effects, showcasing the power of our approach in optimizing protein functionality.
This study represents a significant advancement in the field of computational protein design. By integrating deep learning-driven design with iterative optimization and in silico evaluation, our framework offers a powerful tool for the development of highly functional proteins. The versatility of our platform opens up new avenues for the development of custom-designed proteins across a wide spectrum of applications, from enzyme engineering for industrial biotechnology to the creation of novel biomaterials.
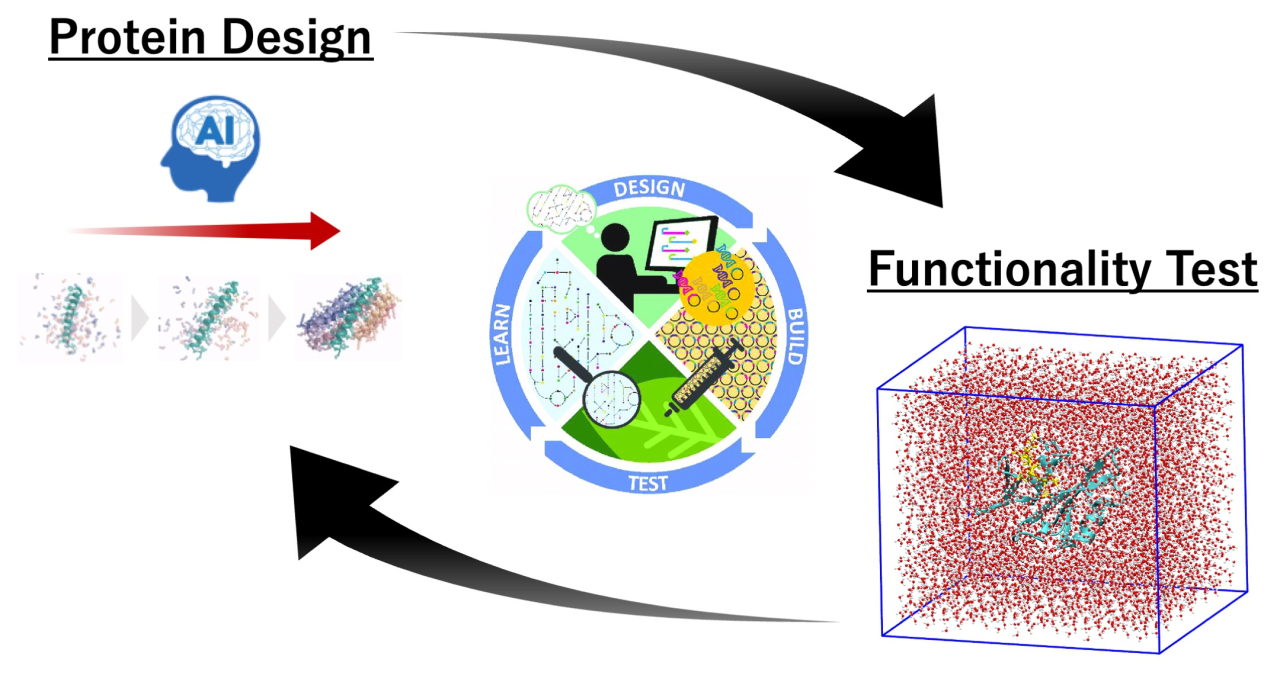