P03-27
Spike separation of high-gamma power in ECoG using peak detection
Masato SAKAGAMI *1, Masashi KINOSHITA2, Mitsutoshi NAKADA2, Kenji SATOU3
1Graduate School of Natural Science and Technology, Kanazawa University
2Department of Neurosurgery, Kanazawa University
3Institute of Transdisciplinary Sciences for Innovation, Kanazawa University
( * E-mail: zdnmasato@stu.kanazawa-u.ac.jp )
Introduction
The brainwaves measured by attaching electrodes directly to the cortical surface are referred to as electrocorticogram (ECoG). Brainwaves exhibit distinct properties based on their frequency ranges. Especially, increase in high-gamma power (HGP) above 60 Hz are often interpreted as indicative of local brain activity associated with specific tasks. In this study, we aimed to analyze the increase of HGP in ECoG data during cognitive tasks within a short time frame of less than 0.1 seconds. Through this process, spike-like signals were frequently found in power above approximately 100 Hz. In noise separation for brainwaves, visual inspection by experts and low-pass filters are commonly used. However, due to the averaging effect of low-pass filters, simple application of them to this case does not work well. Therefore, in this study, we aim to analyze the increase of HGP in more detail by attempting to separate spikes using a method that utilizes peak detection.
Methods
In this study, we used ECoG data from several patients who underwent awake craniotomy at Kanazawa University Hospital in the past. The data were collected while the patients performed specific tasks. The ECoG were measured at a sampling rate of 500 Hz using 16 electrodes arranged in a grid approximately 3 cm square. The cognitive tasks consisted of either a naming task, in which a patient called the name of an illustrated object, or an emotion task, in which a patient identified the emotion shown in a facial photograph.
For preprocessing the data, the following steps were carried out: 1) Perform a Fourier transform on 1-second segments of the brainwave data, with a 0.002-second shift. 2) Perform z-transformation for each frequency greater than 60 Hz to normalize the power across frequencies. 3) Calculate the average across frequencies.
Additionally, for spike separation, the following method was used: 4) Detect peaks of local minimum values in the signal. 5) Since some of the detected peaks have extremely high values far from neighbors, local “maximum” peaks among them are repeatedly detected and removed. 6) Finally, apply spline interpolation to the detected peaks, then reconstruct the signal with the spike components removed.
Results and Discussions
As shown in the figure, this method was able to separate the spike components more appropriately in comparison with the low-pass filter. As a result, the task-related increase in HGP could be identified in more precise time of onset. This suggests that the proposed method may be useful for separating spike components in HGP analysis.
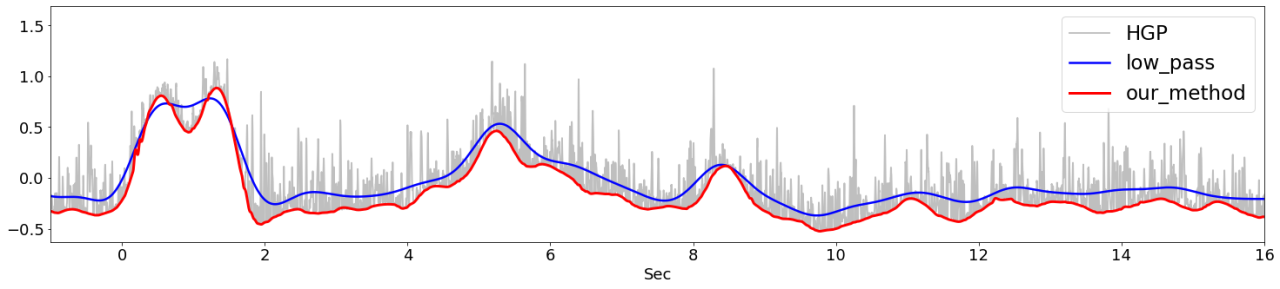