P03-24
Age prediction from DNA methylation data using machine learning
Nagisa MATSUO *1, Kenji SATOU2
1Graduate School of Natural Science and Technology, Kanazawa University
2Institute of Transdisciplinary Sciences for Innovation, Kanazawa University
( * E-mail: matsuo31@stu.kanazawa-u.ac.jp )
Gene expression in living organisms is greatly influenced by the methylation status of DNA. Since the methylation status of DNA changes with age, it is thought that by measuring this, it is possible to estimate a person's biological age (an age that indicates the degree of the body aging, separate from chronological age). In a previous study, Horvarth selected 353 CpG probes from the large number of CpG probes present on DNA to predict biological age, which is highly correlated with chronological age, in various cell types using these methylation levels. In addition, a recent study by Galkin et al. reported that a biological age prediction method using deep learning is effective for large-scale DNA methylation data. In this study, we examine the prediction accuracy of various machine learning algorithms using the same DNA methylation data as in the Galkin’s previous study. Since deep learning does not always achieve the highest accuracy in the field of machine learning that deals with classification and regression problems, it is important to examine how effective other machine learning methods are in predicting biological age from methylation data. As a result of experimenting with four machine learning methods and two importance measures in feature selection, we were able to achieve the highest accuracy (correlation coefficient) of 0.9334 by combining 34 features and random forest. Although the accuracy was slightly lower than the best score 0.94 achieved by previous research using deep learning with 1,000 features, it was confirmed that random forests can also achieve equally accurate predictions with only 34 selected features. Further analysis about the role of these selected CpG probes will be conducted and the results will be presented in poster session.
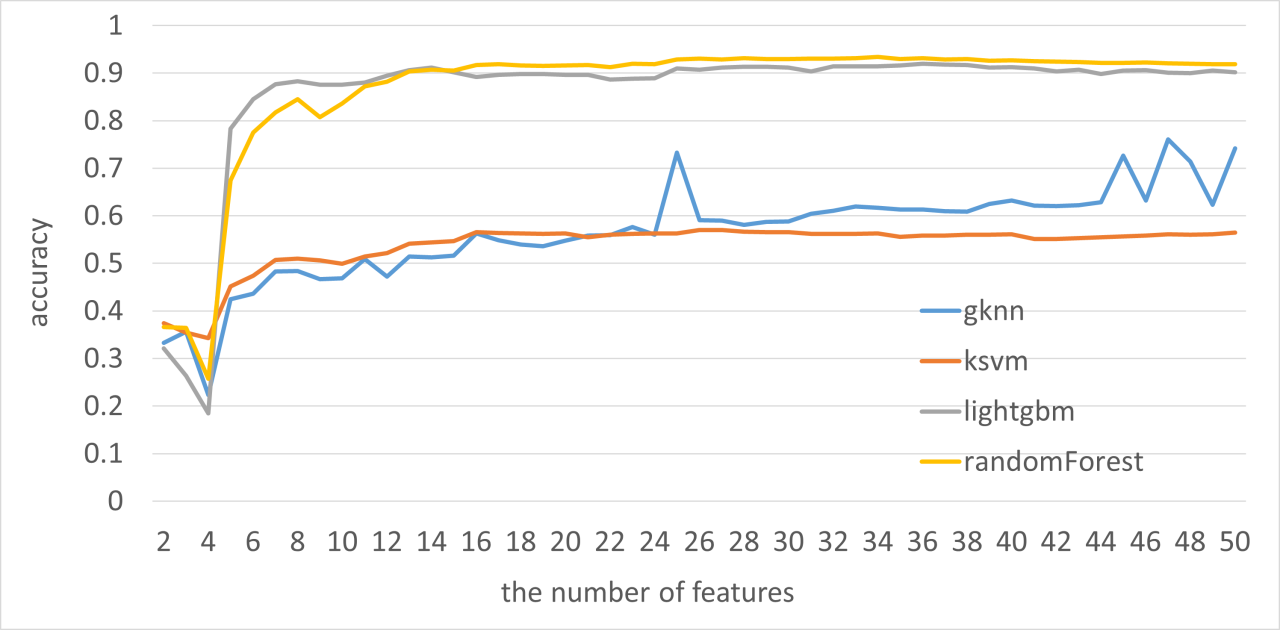