P03-15
Development of the data management system to acquire the strategic data for AI
Miwa SATO *1, Mari OHTA1, Shion HOSODA1, Akira KIMURA2, Takahiro MIMORI2, Michiaki HAMADA2, Daisuke KIGA2, Kazuhide AIKOH1, Miaomei LEI1, Tanabe MAIKO1, Ito KIYOTO1, Akihiko KANDORI1
1Center for Exploratory Research, Research and Development Group, Hitachi, Ltd.
2Department of Electrical Engineering and Bioscience, Faculty of Science and Engineering, Waseda university
( * E-mail: miwa.sato.jr@hitachi.com )
Greenhouse gases are one of the main causes of climate change, and reducing their emissions is a global challenge. To achieve carbon neutrality, we are working on the development of substance production technologies that utilize biological functions. In the development of enzymes responsible for substance production, it is necessary to design their amino acid sequences appropriately. Artificial modification requires many trials due to the complexity of biological functions, so the DBTL cycle is repeated: design the enzyme sequences (Design), build the actual enzyme (Build), evaluate the desired function (Test), interpret the results (Learn), and feed back to the enzyme design again. The DBTL cycle is repeated. Since there are countless combinations of amino acids and the search space is enormous, AI is expected to speed up the process.
However, there are challenges in utilizing AI, such as uniform interpretation of data and information, as well as collection and organization of the large amount of training data required for AI development. In addition, there is still a gap between the number of sequences predicted by the generative AI and the number of sequences that can actually be experimentally verified, making it difficult to experiment with all the sequences predicted by the AI. To effectively proceed with the Build/Test phase of the DBTL cycle, it is necessary to evaluate and select sequences that will be beneficial for AI training.
To solve these data issues, we constructed the data management system that strategically acquires the necessary data for the AI to realize an efficient enzyme development cycle (DBTL cycle) through sequence design by the AI and experimental validation. Issues for improving AI performance were identified and addressed, by building a prototype, we obtained prospects for enzyme improvement through the linkage of AI and wet experiments.
This research is based on results obtained from a project JPNP14004 commissioned by the New Energy and Industrial Technology Development Organization (NEDO).
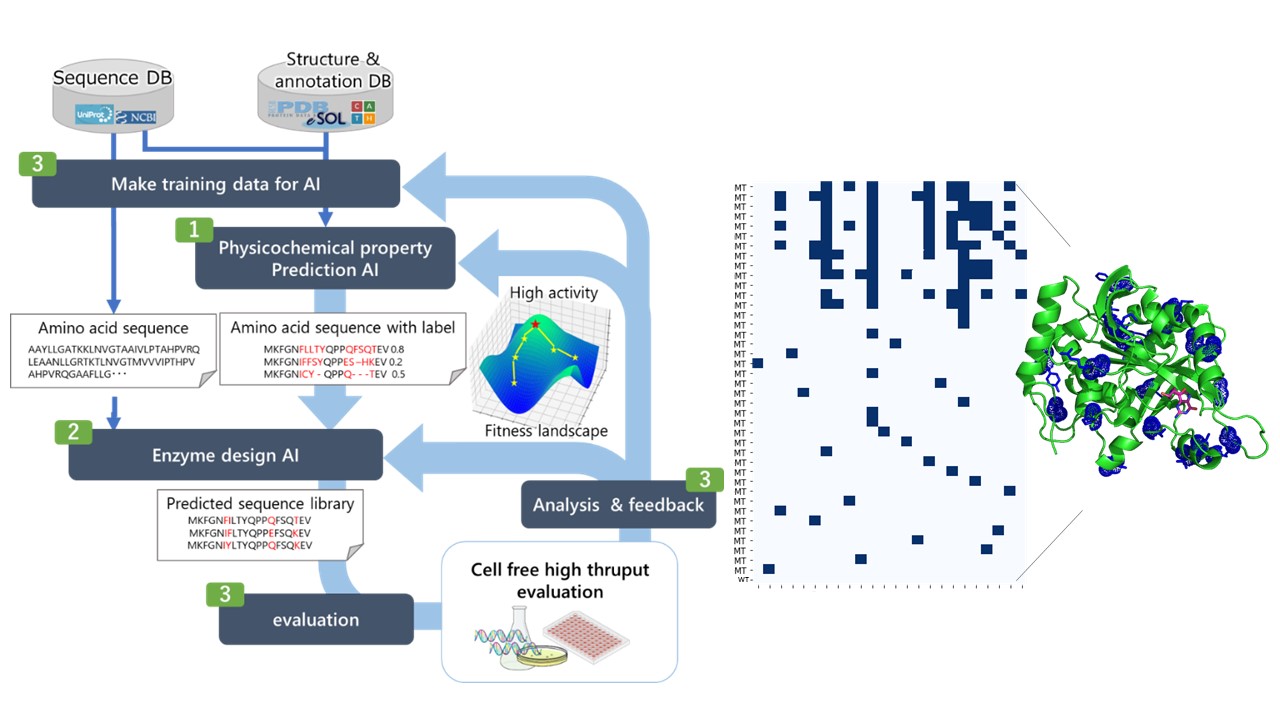