P03-11
CycPeptMP: Development of Membrane Permeability Prediction Model of Cyclic Peptides with Multi-Level Molecular Features and Data Augmentation
Jianan LI *1, Keisuke YANAGISAWA1, 2, Yutaka AKIYAMA1, 2
1Department of Computer Science, School of Computing, Institute of Science Tokyo
2Middle-Molecule IT-based Drug Discovery Laboratory (MIDL), Institute of Science Tokyo
( * E-mail: li@bi.c.titech.ac.jp )
Cyclic peptides are versatile therapeutic agents that boast high binding affinity, minimal toxicity, and the potential to engage challenging protein targets. However, the pharmaceutical utility of cyclic peptides is limited by their low membrane permeability, an essential indicator of oral bioavailability and intracellular targeting. Current machine learning-based models of cyclic peptide permeability show variable performance owing to the limitations of experimental data. Furthermore, these methods only use features derived from the whole molecule that have traditionally been used to predict small molecules and ignore the unique structural properties of cyclic peptides.
We developed CycPeptMP: an accurate and efficient method to predict cyclic peptide membrane permeability [1]. We designed features for cyclic peptides at the atom-, monomer-, and peptide-levels and seamlessly integrated these into a fusion model using deep learning technology. Additionally, we applied various data augmentation techniques to enhance model training efficiency using data from CycPeptMPDB [2], the latest database we constructed. The fusion model exhibited excellent prediction performance for the logarithm of permeability, with a mean absolute error of 0.355 and correlation coefficient of 0.883. Ablation studies demonstrated that all feature levels contributed and were relatively essential to predicting membrane permeability, confirming the effectiveness of augmentation to improve prediction accuracy.
[1] Li J, Yanagisawa K, and Akiyama Y. CycPeptMP: Enhancing Membrane Permeability Prediction of Cyclic Peptides with Multi-Level Molecular Features and Data Augmentation. Brief Bioinform, 2024, accepted.
[2] Li J, Yanagisawa K, Sugita M, Fujie T, Ohue M, and Akiyama Y. CycPeptMPDB: A Comprehensive Database of Membrane Permeability of Cyclic Peptides, J Chem Inf Model, 63(7): 2240–2250, 2023.
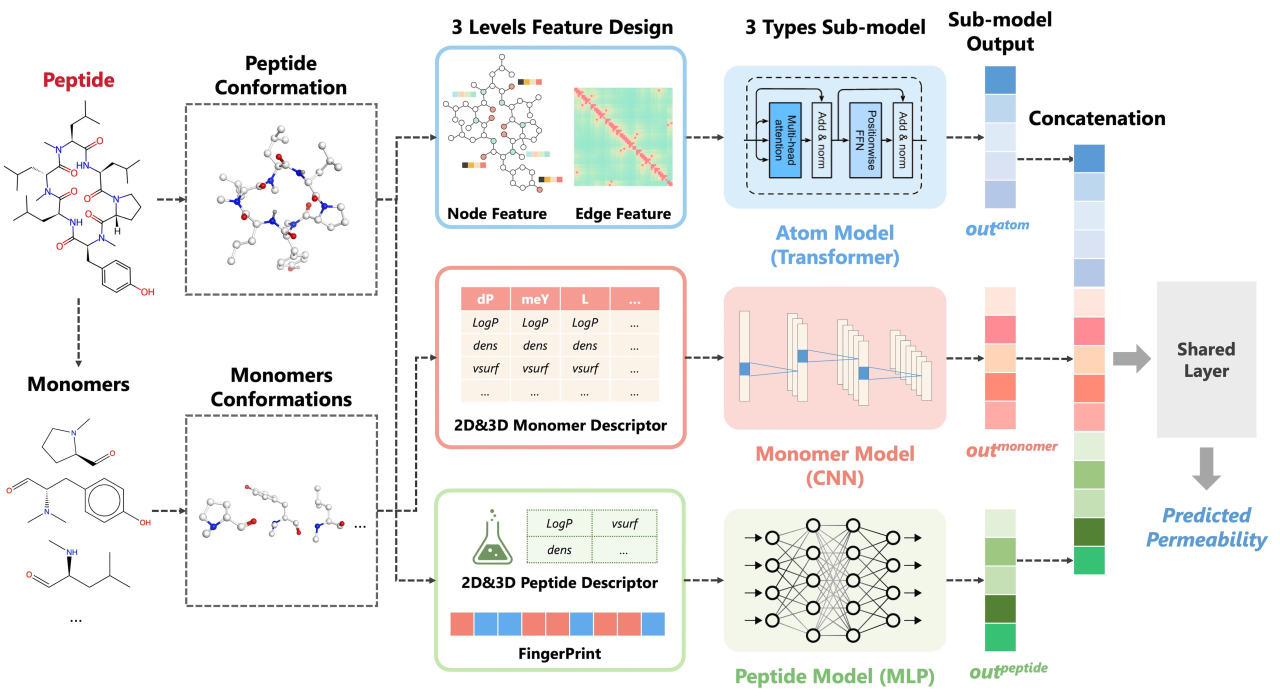