P03-07
Data augmentation method of chimeric protein sequences for fine-tuning of protein language models
Kei YOSHIDA *, Shoji HISADA, Atsushi OKUMA, Taketo KAWARA, Takuya YAMASHITA, Yoshihito ISHIDA, Daisuke ITO, Hiroko HANZAWA, Shizu TAKEDA
Research & Development Group, Hitachi, Ltd.
( * E-mail: kei.yoshida.qp@hitachi.com )
Chimeric antigen receptor-T (CAR-T) cell therapy has produced remarkable clinical responses, especially in cancer treatments. CAR-T cells can recognize and bind specific antigens on the surface of target cells via chimeric antigen receptors (CARs), and finally kill target cells.
CARs are chimeric proteins generated by fusion of functional protein domains which recognize target antigens or perform intracellular signal transduction. Recently, some research groups are trying to optimize CARs to enhance the therapeutic effect of the therapy with computational tools. There are two steps for optimization. First, learning the relationship between protein sequence representations (numerical encodings) and its cellular functions, and second designing new sequences based on the learning results.
Protein language models (PLMs) trained on a huge number of protein sequences are attracting attention as an approach to summarize the protein sequences into representations. In the case of target protein engineering tasks, PLMs fine-tuned on task-specific data have become a powerful tool for getting representations which is useful for state-of-the-art predictive methods. However, there are no PLMs which produce optimal representations from CARs because the sufficient volume of sequence data needed for fine-tuning of PLMs is not available.
In this study, we propose a data augmentation method to increase CAR sequences for efficient fine-tuning of PLMs. In the method, we utilize homologous sequences in each CAR domain and combine them to generate target CAR-like sequence. We fine-tuned ESM-2 using the method and predicted the cytotoxic activity of hundreds of anti-CD19 CAR (28/28/28z) mutants. The results indicate that fine-tuned ESM-2 achieved better predictive performance than the original ESM-2. We conclude that our method will be a reliable approach for representing CAR sequences and lead to designing CARs more efficiently.
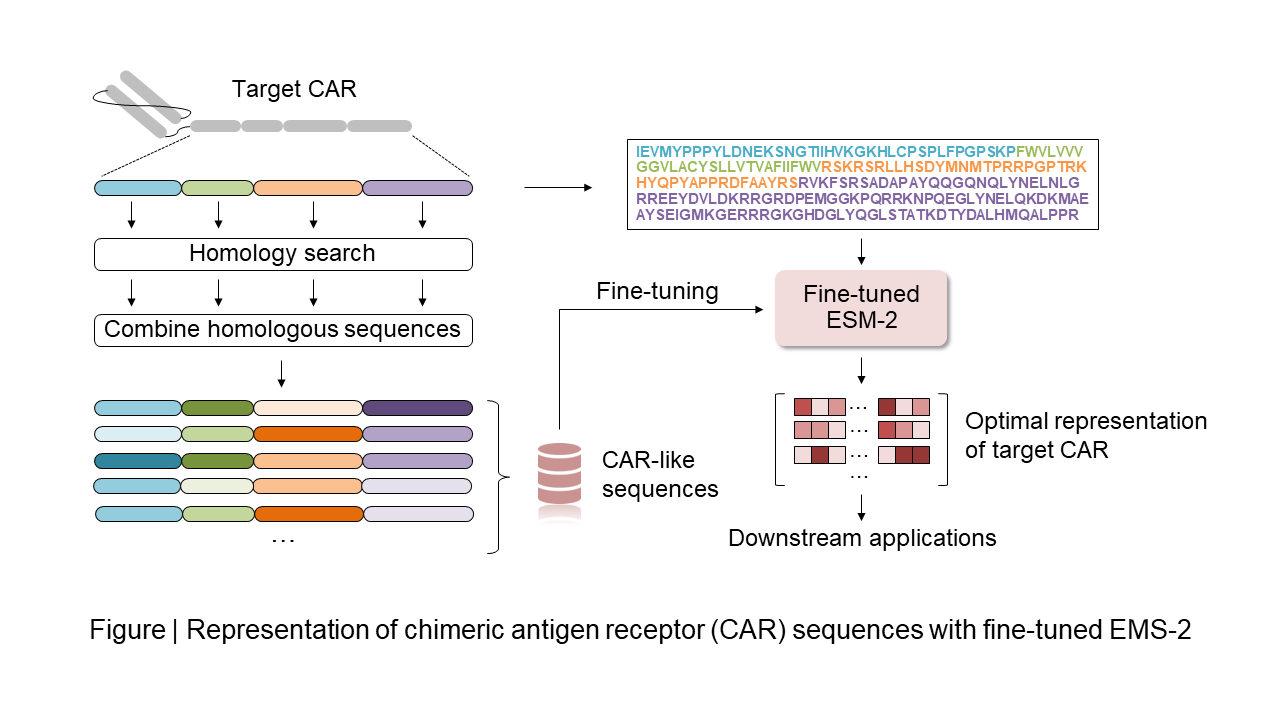