P02-13
Prediction of quantum mechanical interactions between the ligand and each amino acid residue in protein-ligand complexes
Ryosuke KITA *1, Hiromu MATSUMOTO1, Chiduru WATANABE2, Daisuke TAKAYA7, Yu-Shi TIAN7, Masateru OHTA3, Naoki TANIMURA4, Koji OKUWAKI5, Mitsunori IKEGUCHI3, 6, Kaori FUKUZAWA7, Teruki HONMA2, Tsuyohiko FUJIGAYA1, Koichiro KATO1
1Department of Applied Chemistry, Kyushu university
2RIKEN Center for Biosystems Dynamics Research
3RIKEN Center for Computational Science
4Mizuho Research & Technologies, Ltd.
5Engineering Technology Division, JSOL Corporation
6Graduate School of Medical Life Science, Yokohama City University
7Graduate School of Pharmaceutical Sciences, Osaka University
( * E-mail: ryosuke092413@gmail.com )
Evaluating protein-ligand interactions through molecular simulations plays a crucial role in efficiently identifying promising drug candidates from millions of compounds in computational drug discovery. Quantum mechanical (QM) calculations have been expected as an ideal method for evaluating protein-ligand interactions due to their high computational accuracy. However, the high computational cost made it difficult to apply to large molecular systems like proteins. To address this challenge, the fragment molecular orbital (FMO) method was developed, enabling the calculation of protein-ligand interaction energies at the QM level. The FMO method not only allows QM calculations of the entire protein but also enables detailed interaction analysis by extracting protein-ligand interaction energies as inter-fragment interaction energies (IFIE). However, even using the supercomputer Fugaku, FMO method requires several hours of computation time per structure, and further reduction of computational cost has been desired. Therefore, we considered it necessary to develop a machine learning model that predicts IFIE without requiring explicit FMO calculations.
This study aims to develop a machine learning model capable of predicting IFIE between ligands and each amino acid residue constituting the protein within seconds per structure using a standard personal computer. We considered the following methods for descriptors used to numerically represent molecular structures and properties, and machine learning algorithms that use these descriptors as input to predict IFIE between each amino acid residue constituting the protein and the ligand, enabling the model to handle any ligand. For descriptors, we employed Atom-Centered Symmetry Functions (ACSF) [1], a method that can vectorize the surrounding environment of individual ligand constituent atoms. As a machine learning algorithm, we used High-Dimensional Neural Network Potentials (HDNNP) [2], which can learn IFIE as a sum of interactions acting on individual ligand constituent atoms. Although these methods have been developed as methodologies for machine learning force fields, they have not been used for interaction prediction before.
The target protein selected was CDK2. For each of the 80 ligands known to bind to CDK2, 20 binding poses were generated, and descriptors as input were calculated for each amino acid residue (11,9250 points). The model was trained to minimize the error between the IFIE values (true values) calculated using Fugaku and the predicted values. As a result, a trend was observed between the true values and predicted values (Fig. 1), successfully constructing a model capable of rapidly predicting QM interactions between each amino acid residue constituting the protein and the ligand, using 3D atomic coordinates as input.
Reference
[1] J. Behler, J. Chem. Phys. 134, 074106 (2011).
[2] J. Behler, M. Parrinello, Phys. Rev. Lett. 98, 146401 (2007).
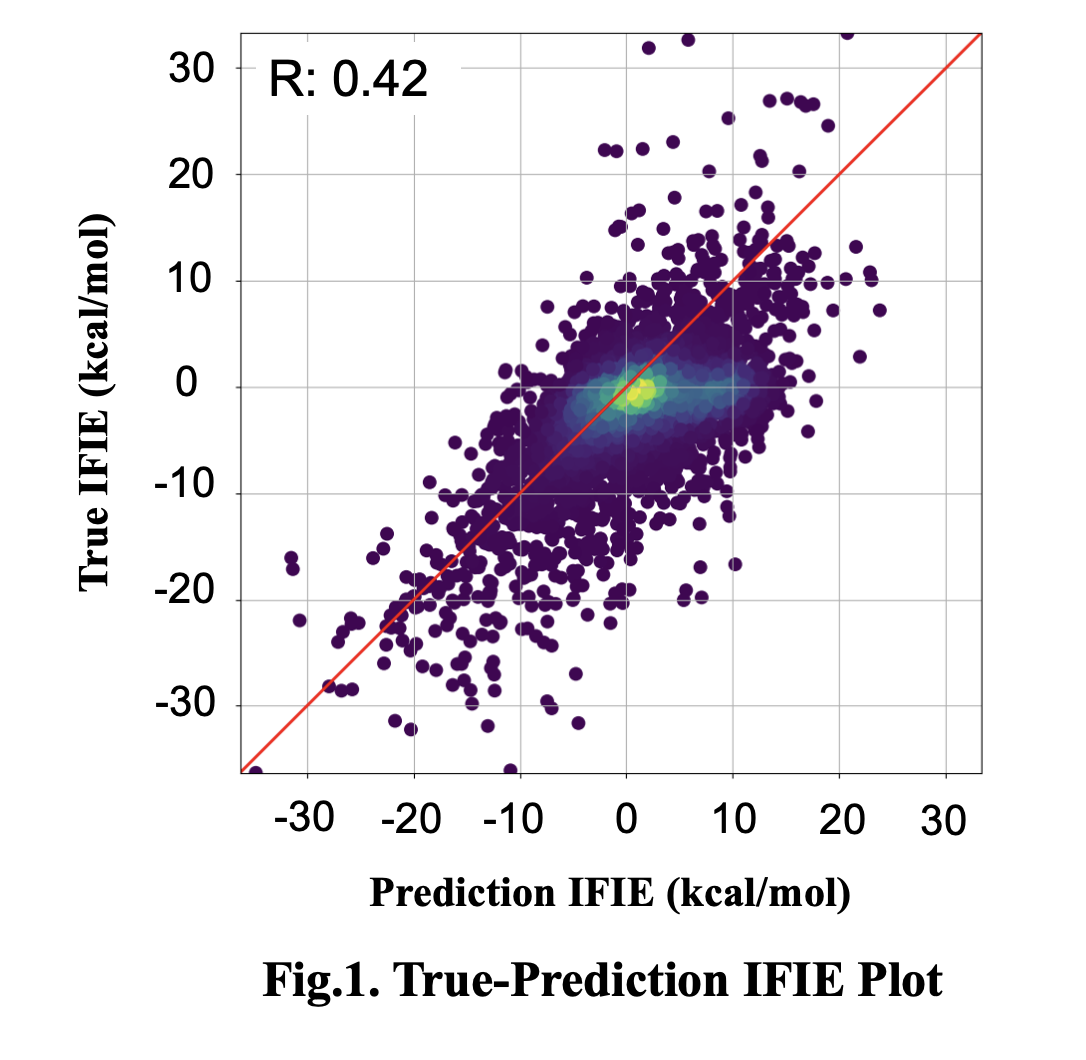