P01-09
Generative Model for Protein Structural Ensembles Enhanced by Molecular Dynamics Simulation Data
Shinji IIDA *1, Yutaka SAITO1, 2, 3
1School of Frontier Engineering, Kitasato University
2Graduate School of Frontier Sciences, The University of Tokyo
3Artificial Intelligence Research Center, AIST
( * E-mail: iida.shinji@kitasato-u.ac.jp )
The function of proteins is closely related to their three-dimensional structure. Proteins recognise other molecules through their three-dimensional structure, performing functions such as catalysing molecular reactions, transport, and transmitting biological signals. The three-dimensional structure of proteins provides useful information for designing molecules that regulate protein function.
Even though static protein structure prediction has become accurate, protein structures fluctuate, and predicting three-dimensional structures whilst considering these fluctuations remains challenging. Molecular dynamics (MD) simulations are known to be an eSective means of studying structural ensembles. However, when applying MD simulations to targets that form diverse structures, they can become trapped in stable states and requires a huge amount of computational time, which makes MD simulations ineSective to obtain a structural ensemble.
To alleviate the eSort of structural ensemble generation, we build a generative model that produces realistic, protein structural ensembles without extensive MD simulations: i. We created training data for structural ensembles by independently performing all-atom MD simulations. ii. We then performed continual pre-training for Pepflow, a diSusion model developed by another group [ Abdin, O.; Kim, P. M. Nat. Mach. Intell. 2024, 1–12.], to expand its applicability domain. While Pepflow primarily used partial structures from PDB as training data, it also incorporated a small amount of MD data. In this study, we expanded the training dataset by conducting MD simulations for 8,000 peptides.
We evaluated the Pepflow models with or without our continual pre-training through the reproducibility of probability distribution with respect to structural quantities of protein structure, such as dihedral angles, bond distance, principal components. For example, Figure 1 indicates a Ramachandran plot of an alanine in a three amino-acid peptide. It demonstrates that the distribution of dihedral angles (middle in Figure 1) was in agreement with that obtained by a MD simulation (left in Figure 1), whereas the distribution of the original Pepflow (right in Figure 1) failed to generate metastable states.
The MD-data enhanced Pepflow would have the potential to improve peptide docking and design and to provide various initial structures of peptides that may enhance the structural sampling coverage of MD simulations.
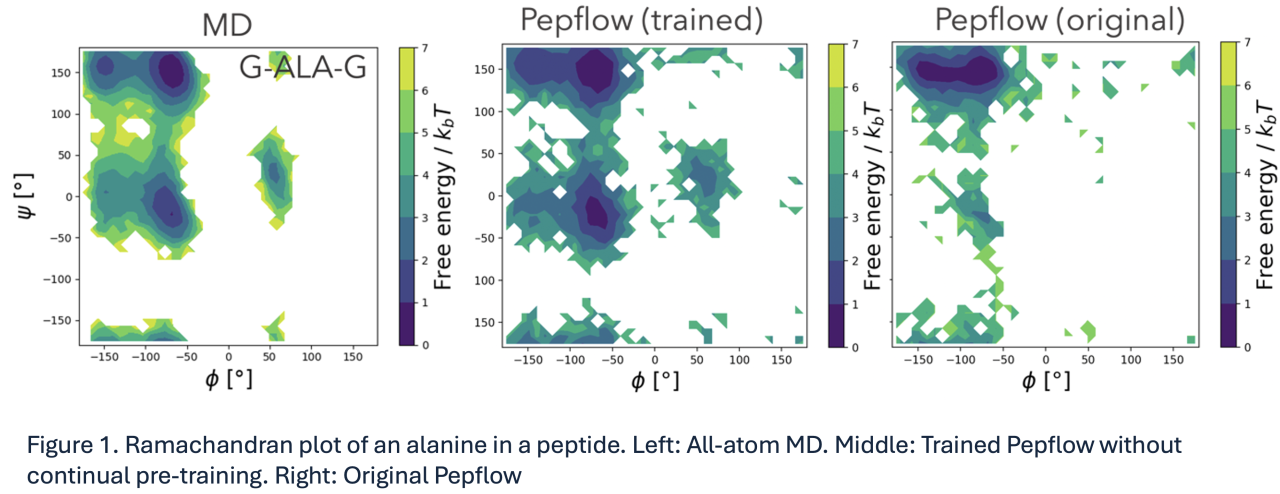