O08_05
Large-Scale MD-Based CPI Prediction Using Supercomputer Fugaku
Natsuki KANAZAWA *1, Shigeyuki MATSUMOTO1, Shuntaro CHIBA2, Yuta ISAKA2, Kiyoshi TAKEMURA2, Mitsugu ARAKI1, Biao MA2, Takao OTSUKA1, Hiroaki IWATA1, Kei TERAYAMA3, Yasushi OKUNO1, 2
1Graduate School of Medicine, Kyoto University
2RIKEN Center for Computational Science
3Graduate School of Medical Life Science, Yokohama City University
( * E-mail: kanazawa.natsuki.82a@st.kyoto-u.ac.jp)
In the early stages of drug discovery, compound screening is conducted to select compounds that interact with target proteins from a vast compound library. To accelerate this process, docking simulations, a computational method based on the 3D structure of proteins, are often employed. However, they typically ignore the dynamics property essential for the protein-compound interaction, resulting in low accuracy for predicting binding poses and affinities.
In contrast, molecular dynamics (MD) simulations are a powerful computational method that accurately capture the dynamic behavior of proteins. MD simulations of compound-protein complexes can calculate binding and dissociation processes and affinities with high precision. Nevertheless, due to the high computational cost, applying MD simulations to large-scale screenings involving thousands to tens of thousands of interactions has been challenging and has not been previously attempted.
In this study, aiming to enable large-scale screenings using MD simulations, we developed a method to evaluate the binding stability of drug candidates through extensive short-time MD calculations using the supercomputer “Fugaku.” This method involves performing short-time MD simulations on tens of thousands of compound-protein complexes generated by docking simulations and comprehensively evaluating their binding stability based on dynamic behavior. Our results demonstrate that it is possible to accurately assess interaction activity with short-time MD calculations on the order of nanoseconds and that this method is applicable to large-scale screenings involving tens of thousands of compounds. Additionally, we developed an automated execution system that allows users with limited computational science expertise to run the entire workflow of this method. In the near future, we aim to further refine this method to develop a practical tool for drug discovery.
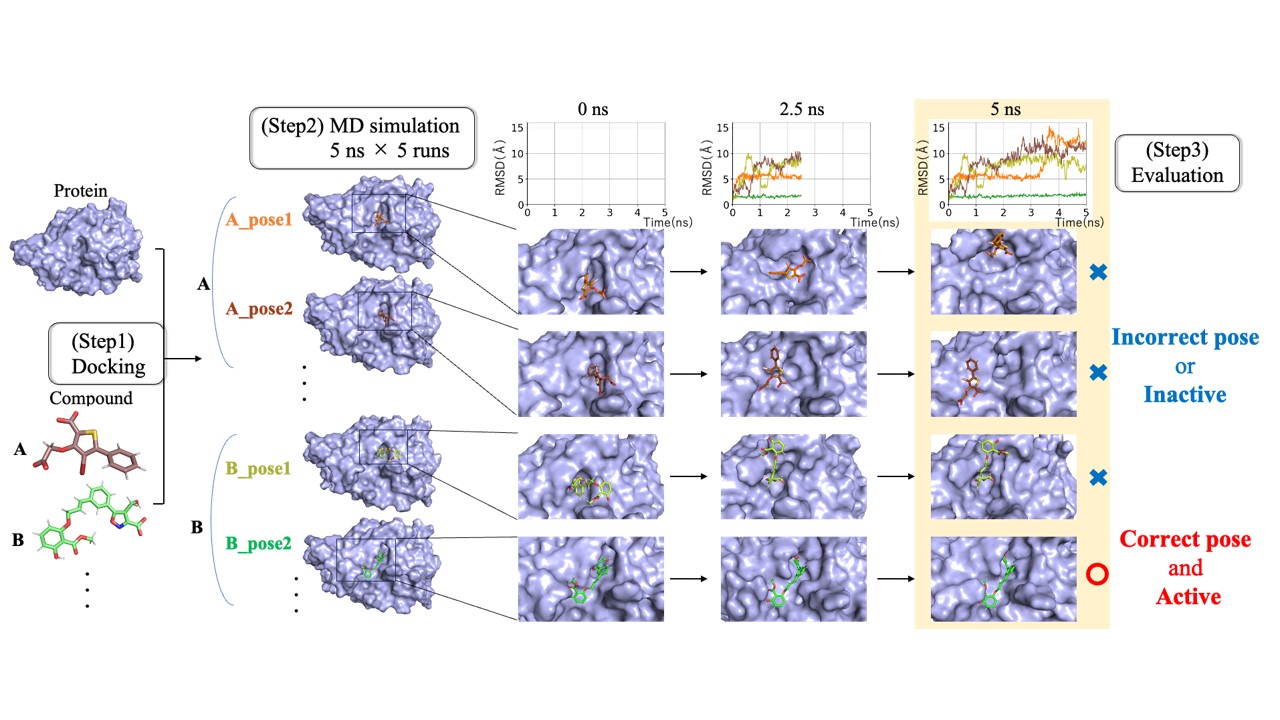