O08_02
Prediction and Analysis of Protein-Ligand Complexes Through R-value Analysis of High-Temperature Molecular Dynamics Simulation
Mochammad Arfin Fardiansyah NASUTION *1, 2, 3, Gert-Jan BEKKER1, Suyong RE3, Chioko NAGAO1, 2, 3, Kenji MIZUGUCHI1, 2, 3
1Laboratory for Computational Biology, Institute for Protein Research, Osaka University
2Department of Chemistry, Graduate School of Science, Osaka University
3Artificial Intelligence Center for Health and Biomedical Research, National Institutes of Biomedical Innovation, Health and Nutrition
( * E-mail: mochammad.arfin@protein.osaka-u.ac.jp )
Docking methods remain the primary approach for predicting protein-ligand interactions but often face reliability issues. While molecular dynamics (MD) simulations can refine docking poses, their high computational cost limits widespread use. To address this issue, we applied a methodology that combines classical docking and high-temperature MD simulations in 10 different enzyme systems, using MOE 2022.10 and GROMACS 2023.3 software, respectively. We utilized R-value-based and protein dynamics analyses to filter and refine the binding configurations produced from docking studies based on ligand binding stability. Our approach found a non-linear relationship between the R-value and RMSD score, where a lower RMSD (indicating similarity to the crystal structure) often corresponds to a better R-value (indicating more stable binding). Notably, 72 out of 98 (73.5%) predicted binding poses that showed the highest stability under 400K MD simulations also had high similarity (RMSD < 3.0 Å). Additionally, our methodology can be used to validate experimental results and explore new potential binding modes. In this study, when applied to the SARS-CoV-2 PLpro system, we found that although 5 out of 8 ligands exhibited high dissimilarity compared to their respective crystal structures, the predicted binding poses retained high stability under our MD simulations. However, the dissimilar regions of the ligand were not well supported by the experimental electron density. Combined, this suggests that the binding modes found by our analysis for these complexes might be the more likely ones. Here, we have shown that our protocol can be used as an effective in-silico approach for analyzing and predicting protein-ligand complexes, without resorting to highly computationally expensive methods such as dynamic docking.
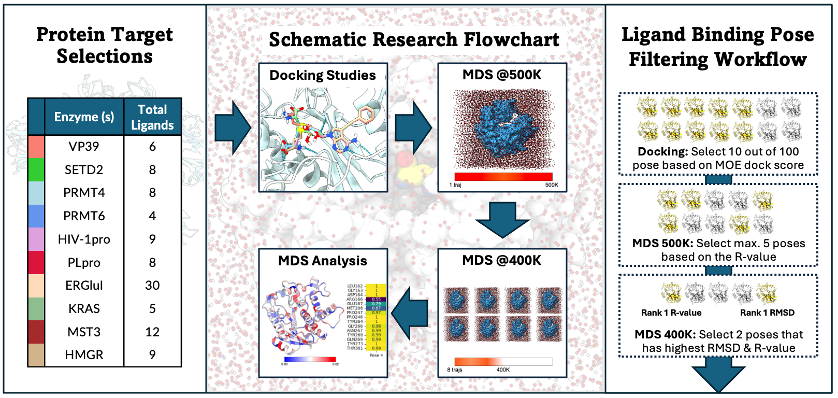