O06_06
Analysis of the usefulness of AlphaMissense score for predicting protein function.
-Evaluation by GLA, the causative gene of Fabry disease-
Yuji SAKAHASHI *1, Yohei MIYASHITA2, 3, Yasuki ISHIHARA2, Osamu YAMAGUCHI1, 4, Yoshihiro ASANO2, 3
1Omics Research Center, National Cerebral and Cardiovascular Center
2BioBank, National Cerebral and Cardiovascular Center
3Department of Genomic Medicine, National Cerebral and Cardiovascular Center
4Department of Cardiology, Pulmonology, Nephrology, and Hypertension, Ehime University
( * E-mail: sakahashi.yuji@ncvc.go.jp)
With the development of next-generation sequencing technology, Variant of Uncertain Significance (VUS) is accumulating. Missense variants, which account for many VUS, tend to have limited training data due to insufficient functional analysis of the mutant protein, making it difficult to create highly accurate in silico pathogenicity prediction models. In this regard, AlphaMissense was announced in 2023 as a new algorithm for predicting the pathogenicity of missense variants. AlphaMissense is a pathogenicity prediction tool based on protein structure information from AlphaFold and has high prediction accuracy for known pathogenic variants. However, because AlphaMissense makes predictions based on protein structural information, its predicted impact on protein function or correlation with clinical phenotypes remain controversial.
In this study, we focused on GLA, the gene responsible for Fabry disease, and evaluated the relationship between the in vitro enzyme activity measurement results of αGAL mutants as a protein function and the AlphaMissense prediction results. From a list of 2,850 GLA variants predicted by AlphaMissense, we used 633 variants for which in vitro enzyme activity data have been published in several previous studies. The enzyme activity of the mutants was evaluated using pctWT, which represents the activity of the αGAL mutant when the activity of the αGAL wild type is taken as 100%. First, the correlation between AlphaMissense score and pctWT was evaluated for 633 variants. The results showed a negative correlation with a correlation coefficient of -0.57. Next, machine learning models were constructed to predict changes in enzyme activity of αGAL mutants using the AlphaMissense score. Three models (Logistic regression, SVM, and XGBoost) were created to classify variants with pctWT reduced to less than 5% as “Severe-LOF” and those with pctWT between 5% and 100 as “Mild-LOF”. To build the models, we used the features from AlphaMissense and the PDB file of αGAL predicted by AlphaFold. The analysis showed that the ROC-AUC for all three models was around 0.8 (Figure 1). These results indicate that the AlphaMissense score is a useful indicator for predicting changes in αGAL enzyme activity. This may be because 1) since αGAL is an enzyme, changes in protein conformation are closely related to changes in enzyme activity, and 2) there is sufficient experimental data on mutants that can be used to build and validate the model. Based on the above, we are currently measuring the enzyme activity of unknown variants of αGAL mutants for which enzyme activity data has not been obtained in previous studies and evaluating the agreement with the model prediction results.
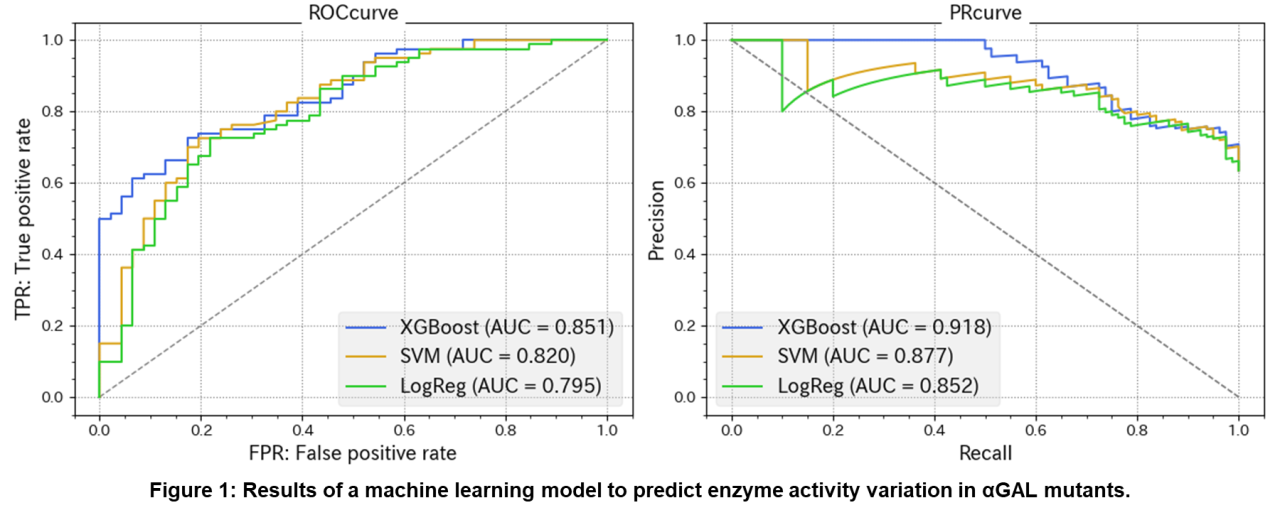