O06_04
Towards the Design of Natural Product Biosynthetic Gene Clusters Using Natural Language Processing Technology
Tomoki KAWANO *1, Taro SHIRAISHI1, 2, Maiko UMEMURA3, Tomohisa KUZUYAMA1, 2
1Graduate School of Agricultural and Life Sciences, The University of Tokyo
2Collaborative Research Institute for Innovative Microbiology, The University of Tokyo
3Bioproduction Research Institute, National Institute of Advanced Industrial Science and Technology
( * E-mail: kawnao-tomoki030@g.ecc.u-tokyo.ac.jp)
Natural products, predominantly synthesized by microorganisms and plants, play crucial roles in modern medicine. These compounds are typically produced by biosynthetic gene clusters (BGCs), which are groups of genes collectively responsible for the synthesis of specific metabolites. With the advent of genome sequencing technologies, a vast amount of genomic data has been accumulated, revealing numerous unknown BGCs.
This study explores the application of natural language processing (NLP) techniques, specifically the RoBERTa algorithm, to understand and generate BGCs. We hypothesized that the evolutionary process of BGCs is recorded in genomic sequences as positional information, analogous to tree rings. By treating functional domains within genes as tokens and gene clusters as sentences, we aimed to capture the relationships between domains using NLP models.
We prepared four datasets of varying complexity: 1) BGCs from the antiSMASH database, 2) complete genomes of Actinobacteria, 3) all bacterial complete genomes, and 4) bacterial and fungal genomes. These datasets were used to train RoBERTa models.
Our results demonstrated that:
1. RoBERTa models successfully learned the relationships between functional domains in BGCs.
2. The models accurately predicted masked domains within known BGCs from the MIBiG database, with over 60% of domains correctly predicted as the top choice and about 90% within the top 10 predictions.
3. The model trained on the most diverse dataset (bacterial and fungal genomes) showed enhanced ability to provide biologically plausible alternatives, potentially useful for exploring novel BGCs.
4. The models could predict the compound class of BGCs with high accuracy, especially for well-studied classes like NRPSs and PKSs.
5. We demonstrated the potential for generating novel BGCs by masking and predicting domains within existing clusters, as exemplified with the cyclooctatin biosynthesis pathway.
Our current models are not specifically trained for BGC generation. As a future direction, we are exploring seq2seq training methods where plausibly less evolved BGCs are used as input to predict more evolved BGCs as output. This approach could lead to the development of models capable of generating evolved BGCs, potentially opening new avenues for the discovery of novel natural products and their biosynthetic pathways.
This study represents a significant step towards leveraging the vast amount of accumulated genomic data for the rational design of novel biosynthetic pathways and the discovery of new natural products, potentially revolutionizing the field of natural product drug discovery.
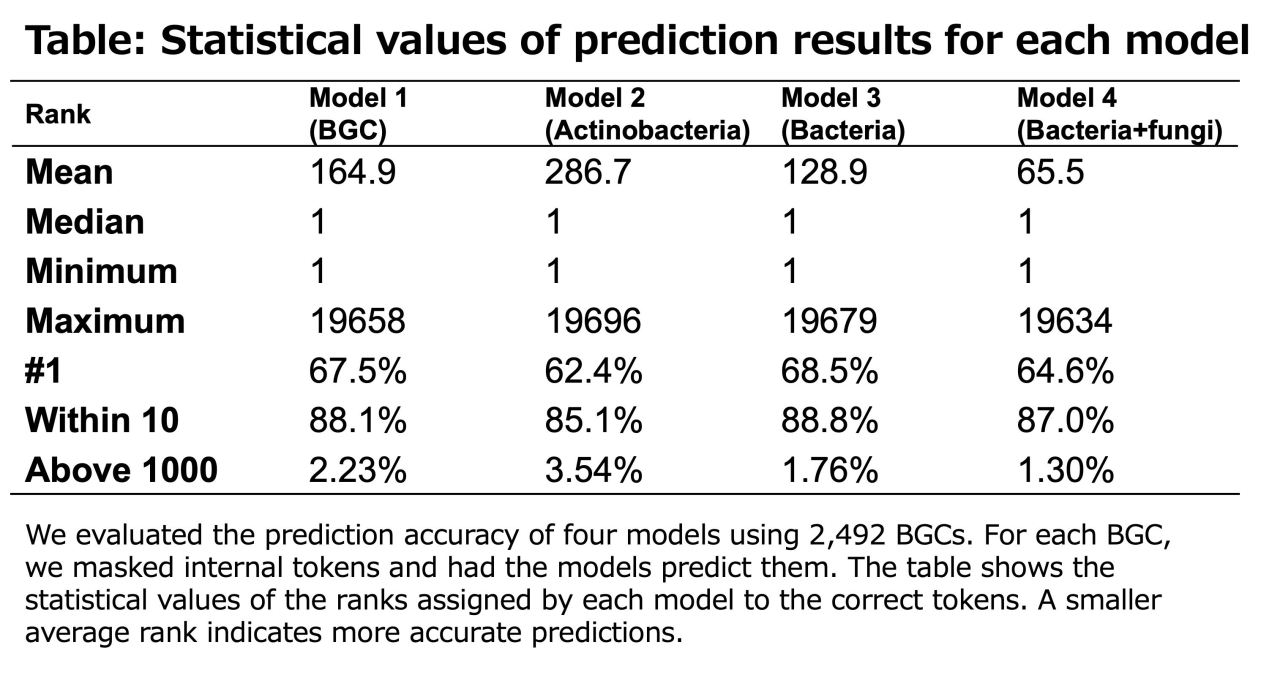