O06_03
Reconstructable latent representation of molecules by Graph Transformer VAE
Yasuhiro YOSHIKAI *, Tadahaya MIZUNO, Hiroyuki KUSUHARA
Laboratory of Molecular Pharmacokinetics, The University of Tokyo Graduate School of Pharmaceutical Sciences
( * E-mail: yoshikai-yasuhiro701@g.ecc.u-tokyo.ac.jp)
Obtaining latent representation of molecules is one of the key processes in data science for chemistry, as it enables various downstream tasks like molecular property prediction.
One of the major representation learning architectures is autoencoder, which learns to encode molecules to latent representations and then decode back to their original structures. This architecture has the advantage that the model can be trained in an unsupervised way, without auxiliary information. However, existing representations like those obtained by unsupervised representation learning have difficulty in restoring the original structure, and existing restorable descriptors, such as those generated by Variational Autoencoder (VAE), have not been well studied about their reconstruction performance.
We first examined the reconstruction performance of molecule of several existing restorable descriptors and found that many of those actually have little reconstruction ability. Besides, the restored molecules often show different molecular properties, such as molecular weight or logP. To address this, we developed a molecular representation which can reconstruct original molecules with high accuracy. Our model is based on VAE, and utilizes graph Transformer in Encoder. The developed representation showed high accuracy in reconstructing the property and structure of original molecules. Notably, we found that decreasing the weight of the KL divergence term of VAE in the reconstruction loss improves the reconstruction performance, while degrading the continuity of the latent space. These results are expected to provide the foundation of unsupervised learning of molecules, and contribute to the improvement and proper usage of restorable latent representation.
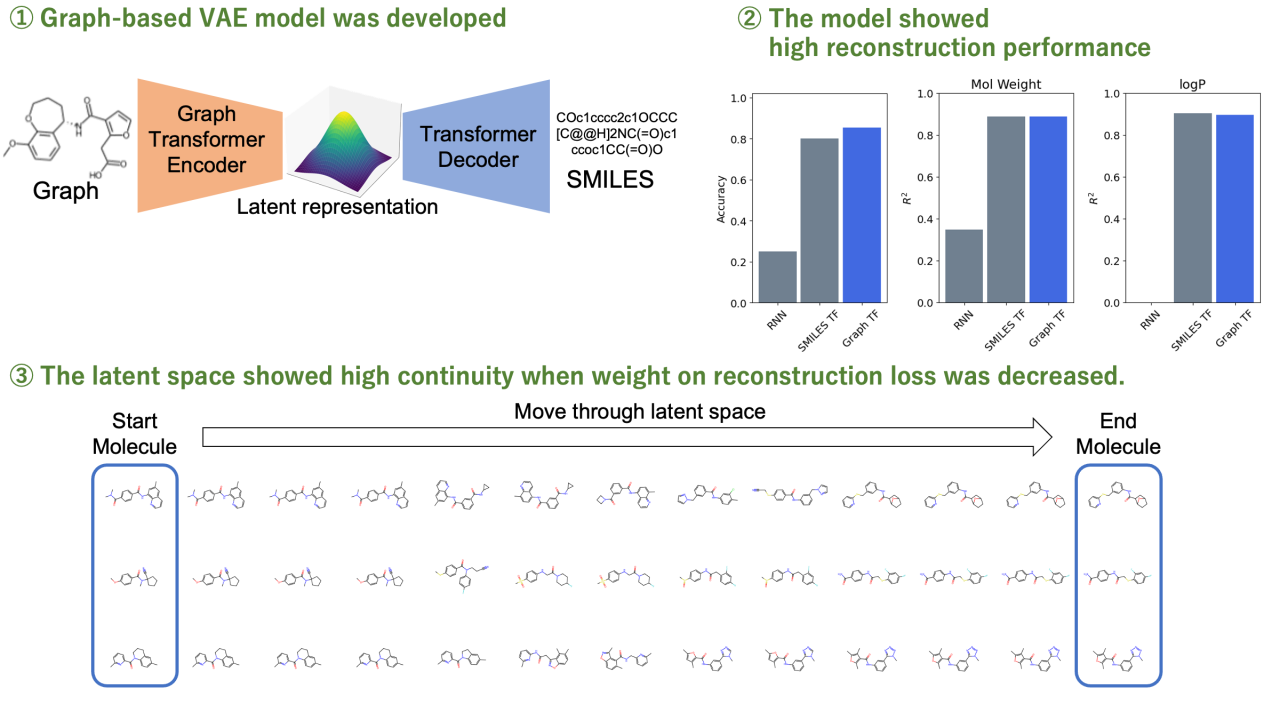