O03_06
Design of Receptor Selective Cell-Penetrating Peptides Using Deep Learning and Simulations
Iori YAMAHATA *1, Hayashi SHUTO2, Koseki JUN3, Shimamura TEPPEI1, 2
1Nagoya University Graduate School of Medicine
2Institute of Science Tokyo
3National Institute of Advanced Industrial Science and Technology
( * E-mail: yamahata.iori.x1@s.mail.nagoya-u.ac.jp)
Despite advances in intracellular drug targeting, the cellular membrane permeability of large molecule drugs remains a major challenge. Cell-penetrating peptides (CPPs) offer promising solutions but are hindered by low cell selectivity. This study developed a novel method to design CPP sequences with enhanced receptor selectivity, focusing on receptor-mediated endocytosis.
Our approach consisted of two main steps:
1. Construction of a deep generative model to create CPP-like sequences:
We developed a CPP-specific model based on EvoDiff, using Low-Rank Adaptation (LoRA). The model was trained on 1,082 known CPP sequences and efficiently generated diverse CPP candidate sequences.
2. An in silico optimization cycle to enhance selectivity:
This cycle, implemented on a supercomputer, involved:
a) Docking simulations between candidate peptides and target receptors
b) Binding energy calculations using molecular dynamics simulations
c) Training of deep learning models to predict binding energies
d) Bayesian optimization for selecting sequences for the next cycle
As a proof of concept, we applied our method to design CPPs that selectively internalize via CXCR4 while minimizing interaction with NRP1. We simulated 2,000 sequences per cycle using DiffDock for molecular docking and MM/GBSA for binding energy estimation.
The optimization cycle progressively improved sequence quality, as indicated by increasing hypervolume metrics. We successfully identified several CPP sequences with high predicted selectivity for CXCR4. In silico validation showed improved binding energies compared to known CPPs.
This computational approach represents a significant advance in the rational design of selective CPPs. The next crucial step will be to validate these findings through in vitro experiments, which will assess the actual receptor selectivity and internalization efficiency of the designed CPPs.
Our method demonstrates the power of combining deep learning, molecular simulations, and optimization techniques in addressing complex biological challenges. Upon experimental validation, this approach could potentially accelerate targeted drug delivery and be applicable to other areas of peptide drug discovery.
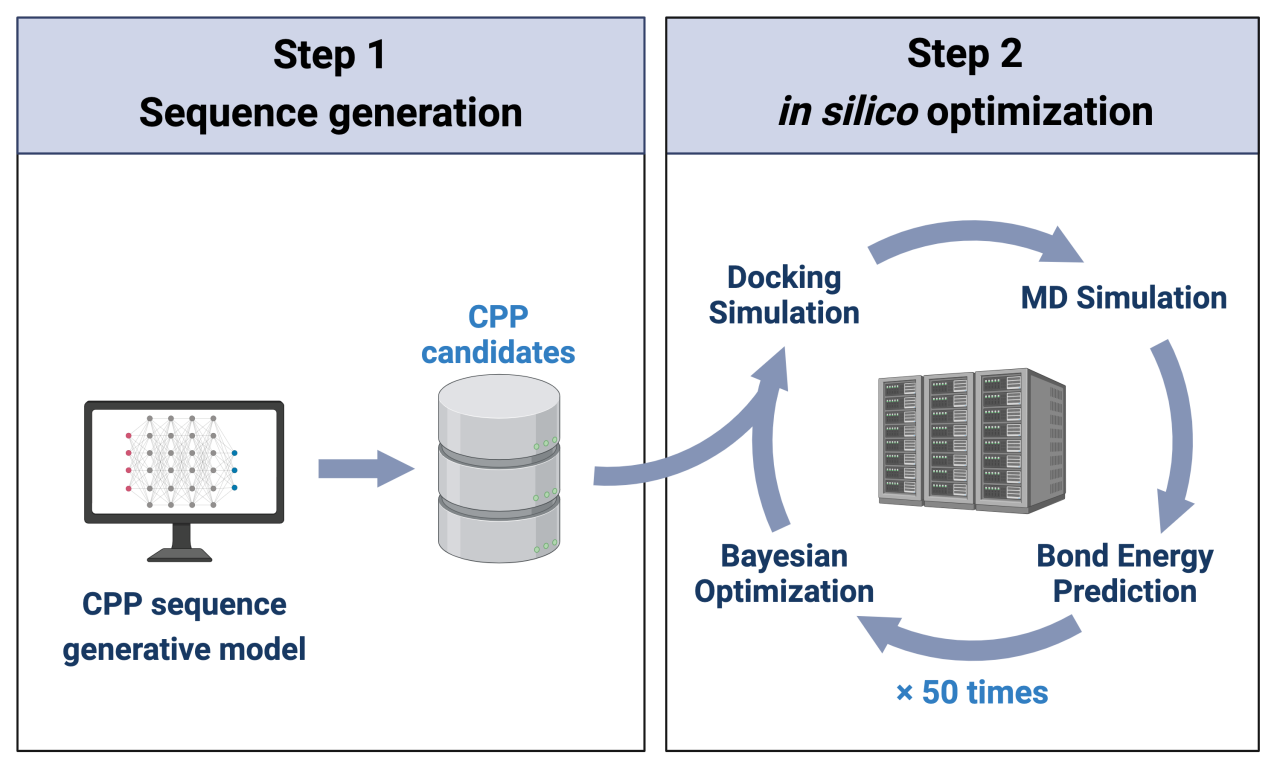