O02_03
Exploring Synthetically Accessible Chemical Spaces with Product-of-Expert Chemical Language Models
Shuya NAKATA *1, Yoshiharu MORI1, 2, Tanaka SHIGENORI1
1Graduate School of System Informatics, Kobe University
2KQCC, Keio University
( * E-mail: nakata@landscape.kobe-u.ac.jp)
Ultra-large synthesis-on-demand chemical spaces have emerged as a valuable resource for drug discovery. Chemical language models that enable direct compound generation have the potential to accelerate the exploration of these vast spaces. However, existing methods are not designed to explore specific chemical spaces and often overlook synthetic accessibility. To address these limitations, we introduce product-of-experts (PoE) chemical language models, a modular and scalable approach to exploring synthetically accessible chemical spaces. This method combines a prior model pre-trained on a chemical space of interest with expert and anti-expert models fine-tuned using external property-specific datasets, allowing for controlled compound generation within the target space.
We constructed an ultra-large chemical space of 1010 compounds for evaluation and found that chemical language models can implicitly learn the underlying generative process, i.e., the possible combinations of building blocks and reactions. Furthermore, we demonstrated that the proposed approach effectively guides compound generation toward desired properties while ensuring synthetic accessibility.
The presentation will detail our method and results, and discuss some implications for future studies.
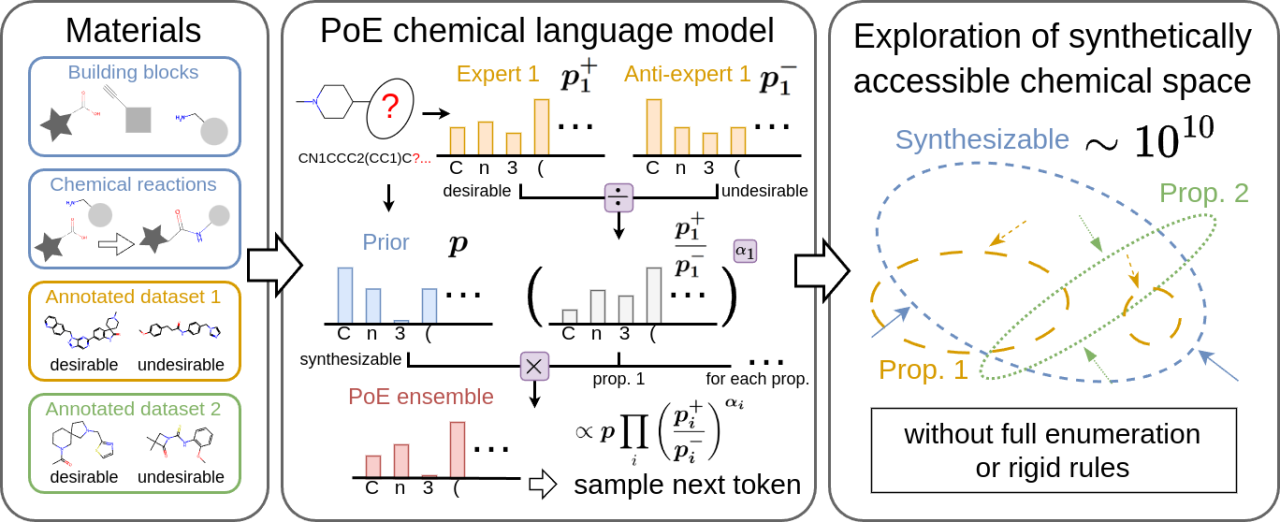