O01_06
Optimizing Multitask Learning with Evolutionary Metrics for Enhanced QSAR-based Natural Product Activity Prediction
Donny RAMADHAN *1, 2, Kenji MIZUGUCHI1
1Laboratory for Computational Biology, Institute for Protein Research, Osaka University
2Research Center for Pharmaceutical Ingredients and Traditional Medicine, National Research and Innovation Agency (BRIN)
( * E-mail: donny.ramadhan@protein.osaka-u.ac.jp)
Natural products exhibit a wide range of structural diversity with their relatively high degree of three-dimensionality, which could play an essential role in their interactions with drug targets. Given the limited availability of bioassay data for pure natural products in public databases, applying multitask learning (MTL) models in quantitative structure-activity relationship (QSAR) studies is expected to enhance the prediction of natural product activity. The effectiveness of transferred information in MTL depends on the relatedness of the tasks combined. However, only a few studies have examined this task-relatedness for use in MTL models, especially for QSAR studies. This research explores the effects of various evolutionary metrics used as input features on the performance of MTL models using limited datasets of natural product biological activities. We curated datasets from the ChEMBL database that comprise the biological activities of natural products against drug targets in the protein kinase group. These datasets were initially filtered using binary classification to identify predicted natural products and their activities. A total of 94 and 86 target proteins were used for classification and regression models, respectively. A single-task learning (STL) model, using Avalon fingerprints (1024 bits) as input features and an artificial neural network, served as the control for predicting the activity of natural compounds for each protein. Subsequently, feature-based multitask learning (FBMTL) was conducted by training the dataset on all proteins within a protein class and predicting the activity of all compounds for each protein. Instance-based multitask learning (IBMTL), a type of FBMTL, incorporated additional input features; in our study, we utilized three types of evolutionary metrics: global sequence similarity, local sequence similarity, and structural similarity of proteins. The results indicate that by leveraging evolutionary relatedness, IBMTL demonstrates statistically significant improvements across all performance parameters of classification and regression models compared to STL, despite using limited datasets of natural products and their bioactivities. FBMTL, on the other hand, fails to show similar improvement in performance.
Keywords: evolutionary relatedness, feature-based multitask learning, instance-based multitask learning, single-task learning
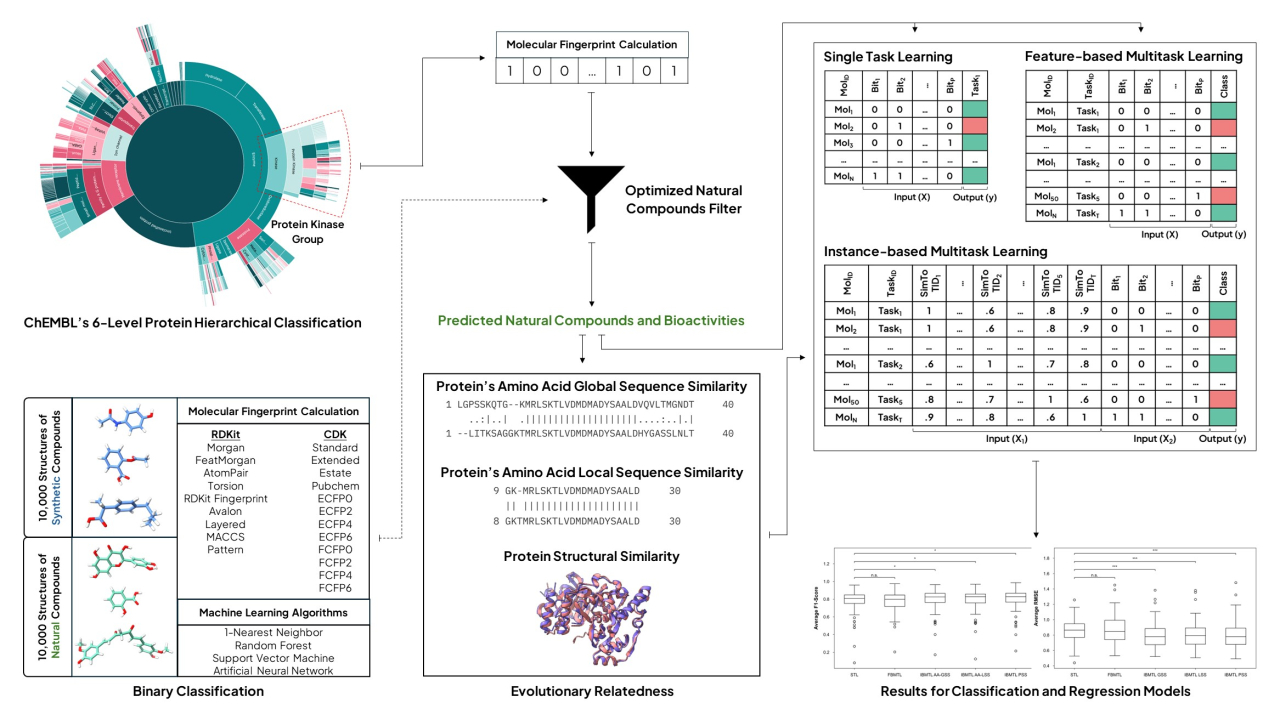